Gillian Hadfield
John Hopkins University
Title: Normative Infrastructure for AI Alignment
Thursday August 8th, 14:00-15:00
Abstract
The challenge of AI alignment has gained increasing importance in recent years as AI systems gain in autonomy and generality. Dominant methods for AI alignment focus on eliciting values, norms and/or preferences from humans and using various methods to train or fine tune models to reflect these elicited values. In this talk I’ll challenge this dominant approach as lacking robustness, legitimacy, feasibility and, most importantly, a realistic relationship to how human normative systems have evolved and how they function. I’ll explain the role of normative infrastructure—the institutions, behaviors and cognitive processes that’s secure normative social order in human societies. I’ll outline an alternative approach to alignment and present early MAS results that focus on endowing AI agents and environments with normative infrastructure to align more effectively and robustly with human normativity.
Bio
Gillian K. Hadfield is an economist, legal scholar and a computational social scientist pondering how humans build the normative world and how to make a world with AI better for all. She has recently joined Johns Hopkins University, where she is jointly appointed to the faculties of the School of Government and Policy and of the Department of Computer Science at the Whiting School of Engineering. Hadfield is also a Canada CIFAR AI Chair at the Vector Institute for Artificial Intelligence and a Schmidt Sciences AI2050 Senior Fellow. Hadfield’s research is focused on innovative design for legal and regulatory systems for AI and other complex global technologies; computational models of human normative systems; and working with machine learning researchers to build ML systems that understand and respond to human norms. She is a faculty affiliate at the Center for Human-Compatible AI at the University of California Berkeley and she was previously the inaugural Director (2019-2023) and Chair (2019-2024) of the Schwartz Reisman Institute for Technology and Society at the University of Toronto, and a senior policy advisor at OpenAI. Her book Rules for a Flat World: Why Humans Invented Law and How to Reinvent It for a Complex Global Economy was published by Oxford University Press in 2017; a paperback edition with a new prologue on AI was published in 2020 and an audiobook version released in 2021.
Winner of the IJCAI 2024 Award for Research Excellence
Thomas Dietterich
Oregon State University
Title: AI in Open Worlds: A Progress Report
Thursday August 8th, 9:00-10:00
Abstract
In 2008, Eric Horvitz titled his AAAI Presidential Address “Artificial Intelligence in the Open World” in which he raised the challenge of creating AI systems that can operate successfully in open worlds. He pointed out that from the earliest days, AI researchers have worried about this challenge and yet virtually all AI research has assumed closed worlds. This talk will summarize the (modest) progress that we have made since 2008 and the many challenges that remain wide open. What is required of our AI architectures and human-computer interfaces to create human+computer systems that are–in the words of David Woods–poised to adapt?
Bio
Dr. Dietterich (AB Oberlin College 1977; MS University of Illinois 1979; PhD Stanford University 1984) is Distinguished Professor Emeritus in the School of Electrical Engineering and Computer Science at Oregon State University. Dietterich is one of the pioneers of the field of Machine Learning and has authored more than 220 refereed publications and two books. His current research topics include novelty-aware artificial intelligence, robust human-AI systems, and applications in sustainability.
Dietterich has devoted many years of service to the research community and recently received the ACML Distinguished Contribution and the AAAI Distinguished Service awards. He is a former President of the Association for the Advancement of Artificial Intelligence and the founding president of the International Machine Learning Society. Other major roles include Executive Editor of the journal Machine Learning, co-founder of the Journal for Machine Learning Research, and program chair of AAAI 1990 and NIPS 2000. He currently oversees the Computer Science categories at arXiv.
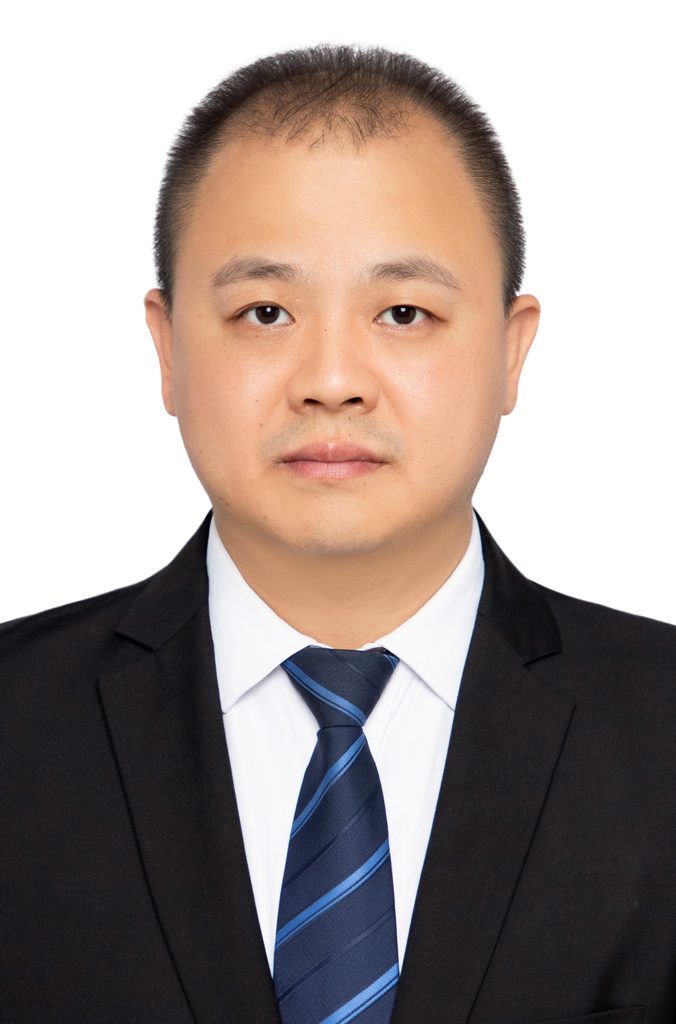
Bo An
Nanyang Technological University, Singapore
Title: From Algorithmic and RL-based to LLM-powered Agents
Wednesday August 7th, 14:00-15:00
Abstract
In the early days of tackling AI problems involving complex cooperation and strategic interactions, algorithmic approaches were widely employed. Reinforcement learning has since proven effective in learning efficient policies for large-scale optimization problems that are beyond the scalability of traditional algorithmic approaches. Recently, the use of large language models (LLMs) as computational engines has given rise to a new paradigm: LLM-powered agents capable of addressing complex problems across various domains. This talk will explore our recent work within these three paradigms and offer insights into the development of scalable, efficient, and distributed artificial general intelligence.
Bio
Bo An is a President’s Chair Professor at Nanyang Technological University, Singapore. He received the Ph.D degree in Computer Science from the University of Massachusetts, Amherst. His current research interests include artificial intelligence, multiagent systems, computational game theory, and reinforcement learning. Dr. An was the recipient of the 2010 IFAAMAS Victor Lesser Distinguished Dissertation Award, an Operational Excellence Award from the Commander, First Coast Guard District of the United States, the 2012 INFORMS Daniel H. Wagner Prize for Excellence in Operations Research Practice, 2018 Nanyang Research Award (Young Investigator), and 2022 Nanyang Research Award. His publications won the Best Innovative Application Paper Award at AAMAS’12, the Innovative Application Award at IAAI’16, and the best paper award at DAI’20. He was invited to give Early Career Spotlight talk at IJCAI’17. He led the team HogRider which won the 2017 Microsoft Collaborative AI Challenge. He was named to IEEE Intelligent Systems’ “AI’s 10 to Watch” list for 2018. He was PC Co-Chair of AAMAS’20 and General Co-Chair of AAMAS’23. He will be PC Chair of IJCAI’27. He is a member of the editorial board of JAIR and is the Associate Editor of AIJ, JAAMAS, IEEE Intelligent Systems, ACM TAAS, and ACM TIST. He was elected to the board of directors of IFAAMAS, senior member of AAAI, and Distinguished member of ACM.
Winner of the Computers and Thought Award
Nisarg Shah
University of Toronto, Canada
Title: Democratic Foundations of Fair AI via Social Choice
Wednesday August 7th, 9:00-10:00
Abstract
Over the millennia, human society has invented numerous systems for decision-making, from early councils and monarchies to modern democratic systems, ensuring that these systems treat individuals and groups fairly. With AI now emerging as the latest decision-making tool, there is naturally a growing interest in ensuring the fairness of AI-driven decisions. In this talk, I will present an approach to algorithmic fairness inspired by democratic principles. First, I will review mathematical treatments of this approach in computational social choice, with applications to elections and resource allocation. Then, I will talk about how this framework can be extended to AI applications, such as classification, clustering, and reinforcement learning, providing broadly applicable criteria that are less sensitive to group definitions. Finally, I will discuss how this approach can be applied to AI alignment more broadly, and how robustness mechanisms designed for democratic governance systems can be adapted to the AI ecosystem.
Bio
Nisarg Shah is an Associate Professor of Computer Science at the University of Toronto. He is also a Research Lead for Ethics of AI at the Schwartz Reisman Institute for Technology and Society, a Faculty Affiliate of the Vector Institute for Artificial Intelligence, and an Advisor to the nonprofit AIGS Canada. His distinctions include the Computers and Thought Award (IJCAI, 2024), the Kalai Prize (Game Theory Society, 2024), Innovators Under 35 (MIT Technology Review Asia Pacific, 2022), AI’s 10 to Watch (IEEE Intelligent Systems, 2020), Victor Lesser Distinguished Dissertation Award (IFAAMAS, 2016), and PhD Fellowship (Facebook, 2014-15). His research develops theoretical foundations for algorithmic fairness across a range of domains including voting, resource allocation, matching, and machine learning. He has co-developed the not-for-profit website Spliddit.org, which has helped more than 250,000 people make provably fair decisions in their everyday lives. He earned his PhD in computer science at Carnegie Mellon University and was a postdoctoral fellow at Harvard University.
Winner of the John McCarthy Award
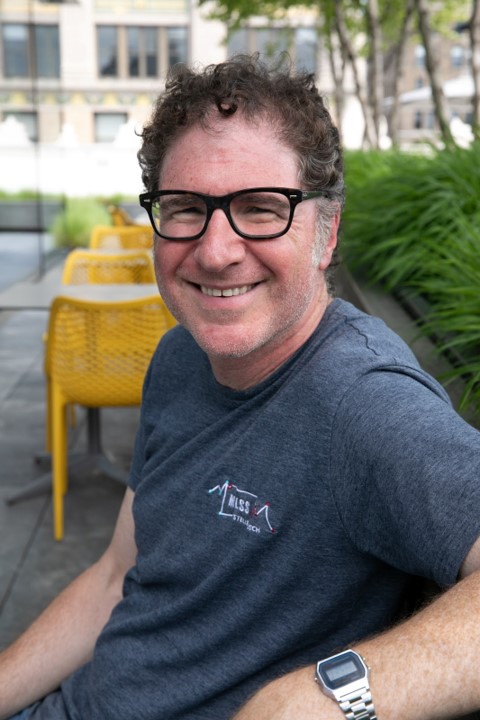
David Blei
Columbia University
Title: Beyond Roll Call: Inferring Politics from Text
Tuesday August 6th, 11:30-12:30
Abstract
The ideal point model is a staple of quantitative political science.
It is a probabilistic model of roll call data—how a group of lawmakers
vote on a collection of bills—that can be used to quantify the
lawmakers’ political positions, which are called ‘ideal points.’ In
this talk, I will discuss two ways to incorporate political texts into
ideal point models. One source of text is the collection of bills. The
issue-adjusted ideal point model helps capture how a lawmaker’s
political position might change depending on the content of the bill
under consideration. It helps find sensible multi-dimensional ideal
points, which are difficult to estimate from the votes alone. Another
source of text comes from the lawmakers. In addition to voting,
lawmakers express their political positions through speeches, press
statements, and tweets. The text-based ideal point model can be used
to analyze a collection of texts to quantify the political positions
of their authors. It helps find ideal points for anyone who authors
political texts, including non-voting actors like candidates and
political commentators.
(This is joint work with Sean Gerrish, Keyon Vafa and Suresh Naidu)
Bio
David Blei is a Professor of Statistics and Computer Science at
Columbia University, and a member of the Columbia Data Science
Institute. He studies probabilistic machine learning and Bayesian
statistics, including theory, algorithms, and application. David has
received several awards for his research. He received a Sloan
Fellowship (2010), Office of Naval Research Young Investigator Award
(2011), Presidential Early Career Award for Scientists and Engineers
(2011), Blavatnik Faculty Award (2013), ACM Prize in Computing (2013),
a Guggenheim fellowship (2017), a Simons Investigator Award (2019),
the Lenfest Distinguished Faculty Award (2024), the AAAI John McCarthy
award (2024), and the ACM/AAAI Allan Newell Award (2024). He was the
co-editor-in-chief of the Journal of Machine Learning Research from
2019-2024. He is a fellow of the Association for Computing Machinery
(ACM) and the Institute of Mathematical Statistics (IMS).
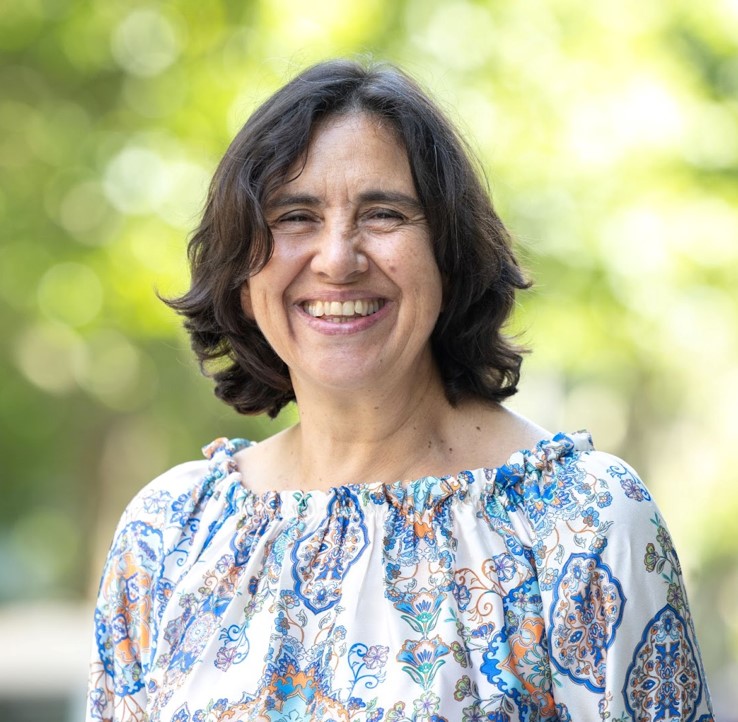
Francesca Toni
Imperial College, United Kingdom
Title: Arguing with Machines: Bridging Explainable AI and Contestability
Friday August 9th, 9:00-10:00
Abstract
AI has become pervasive in recent years, and the need for explainability is widely agreed upon as crucial for safe and trustworthy deployment of AI systems. However, state-of-the-art AI and eXplainable AI (XAI) approaches mostly neglect the need for AI systems to be contestable, as advocated instead by AI guidelines (e.g. by the OECD) and regulation of automated decision-making (e.g. GDPR in the EU and UK). In this talk I will explore how contestability can be achieved computationally, starting from various approaches to explainability, mostly drawn from the field of computational argumentation. This is a well-established branch of AI for automating ‘machine arguing’ based on (i) abstractions (termed argumentation frameworks), representing information in terms of arguments and dialectical relations between them, and (ii) evaluation methods (termed semantics) for reaching consensus regarding conclusions to be drawn. I will advocate computational argumentation as ideally suited to support contestable AI that can (1) interact to progressively explain outputs and/or reasoning as well as assess grounds for contestation provided by humans and/or other machines, and (2) revise decision-making processes to redress any issues successfully raised during contestation. Specifically, I will overview a number of approaches to argumentation-based XAI for opaque and transparent forms of AI and their uses to achieve contestability.
Bio
Francesca Toni is a Professor in Computational Logic and Royal Academy of Engineering/JP Morgan Research Chair on Argumentation-based Interactive Explainable AI (XAI) at the Department of Computing, Imperial College London, UK, as well as the founder and leader of the CLArg (Computational Logic and Argumentation) research group and of the Faculty of Engineering XAI Research Centre. She holds an ERC Advanced grant on Argumentation-based Deep Interactive